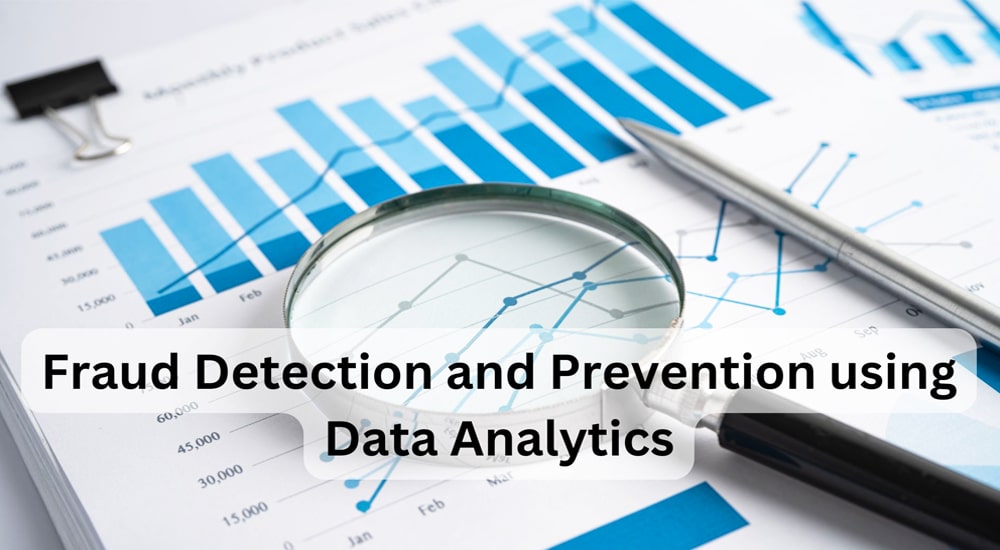
Introduction to Fraud Detection and Prevention
Fraud detection and prevention are critical for safeguarding organizations and individuals against financial losses, identity theft, and other fraudulent activities. Fraud can manifest in various forms, including financial fraud, cyber fraud, and identity theft, each posing significant risks to both private and public sectors. The financial impact of fraud can be substantial, affecting not only the immediate finances but also long-term reputations and operational integrity.
Data analytics has emerged as a powerful tool in the fight against fraud. By leveraging data-driven insights, organizations can detect fraudulent activities more accurately and swiftly. The role of data analytics in fraud detection is multifaceted: it involves analyzing transaction data, user behavior, and historical patterns to identify anomalies and suspicious activities. This proactive approach allows for early detection of potential fraud, thereby reducing the risk of substantial financial losses.
Key Components of Fraud Detection Systems
Fraud detection systems rely on several key components to effectively identify and prevent fraudulent activities. Understanding these components is crucial for building robust fraud prevention strategies.
Types of Fraud: Fraud can be categorized into several types, including financial fraud (e.g., embezzlement, credit card fraud), identity theft (e.g., stolen personal information), and cyber fraud (e.g., phishing attacks, ransomware). Each type requires specific detection methods and preventive measures.
Data Sources: Effective fraud detection systems integrate various data sources, such as transaction records, user behavior logs, and historical fraud data. By analyzing these data points, organizations can detect unusual patterns and flag potentially fraudulent activities.
Detection Techniques: Several analytical techniques are employed in fraud detection. Anomaly detection identifies deviations from normal behavior, such as unusual transaction patterns. Pattern recognition involves analyzing historical data to identify common fraud patterns. Additionally, predictive modeling uses statistical and machine learning techniques to forecast potential fraud based on historical data.
Together, these components form the backbone of a comprehensive fraud detection system. By leveraging advanced data analytics, organizations can enhance their ability to detect and prevent fraud, protecting themselves from significant financial and reputational damage.
Data Collection and Integration
Effective fraud detection relies on comprehensive data collection and integration from various sources. Data collection involves gathering relevant information that can reveal fraudulent activities. Common data sources include transaction records, user account information, behavioral data, and external data such as credit scores and public records. For instance, transaction data may include details about purchase amounts, locations, and timestamps, while behavioral data encompasses patterns in user activity and interactions.
Challenges in Data Integration often arise from combining data from disparate sources. Different systems may store data in various formats and structures, making it difficult to unify and analyze. Ensuring consistency and accuracy across these datasets is crucial for effective fraud detection. Techniques such as data normalization and data cleansing are used to address these challenges, ensuring that integrated data is reliable and usable.
Importance of Data Quality cannot be overstated. High-quality data is essential for accurate analysis and effective fraud detection. Incomplete, outdated, or incorrect data can lead to false positives or negatives, undermining the reliability of fraud detection systems. Implementing rigorous data validation processes and maintaining up-to-date records are key practices in ensuring data quality.
Analytical Techniques for Fraud Detection
Fraud detection leverages various analytical techniques to identify and prevent fraudulent activities. Statistical Methods such as regression analysis and clustering are commonly used. Regression analysis helps identify relationships between variables and detect anomalies, while clustering groups similar data points to identify outliers that may indicate fraud.
Machine Learning Algorithms play a crucial role in modern fraud detection. Techniques such as decision trees, neural networks, and ensemble methods are employed to analyze complex datasets and identify patterns indicative of fraud. For example, decision trees can classify transactions as fraudulent or legitimate based on learned criteria, while neural networks can detect subtle, non-linear patterns in large datasets.
Real-Time vs. Batch Processing is another critical aspect. Real-time processing involves analyzing data as it is collected, allowing for immediate detection of fraudulent activities. Batch processing, on the other hand, involves analyzing accumulated data at scheduled intervals. Both approaches have their applications, with real-time processing being essential for immediate fraud detection and batch processing useful for comprehensive, periodic analysis.
By combining these analytical techniques, organizations can enhance their fraud detection capabilities, improving their ability to identify and mitigate fraudulent activities effectively.
Fraud Prevention Strategies
Preventing fraud involves implementing proactive measures to reduce the risk of fraudulent activities. Employee Training is a fundamental strategy, as educating staff about recognizing and reporting suspicious behavior can prevent internal fraud and improve overall vigilance.
Access Controls are another critical component. Implementing robust access management systems ensures that only authorized individuals can access sensitive information and systems. Multi-factor authentication and regular audits of access permissions are effective practices in maintaining security.
Data Analytics also plays a vital role in fraud prevention. By analyzing historical data and identifying common fraud patterns, organizations can develop preventive measures tailored to their specific risks. For example, setting thresholds for transaction amounts or flagging unusual behavior based on past data can help in preemptively addressing potential fraud.
Technology Integration is crucial for comprehensive fraud prevention. Leveraging tools such as fraud detection software, risk management platforms, and cybersecurity solutions can enhance an organization’s ability to prevent and respond to fraud effectively.
In summary, combining proactive strategies, employee training, and advanced data analytics helps organizations to not only detect but also prevent fraudulent activities, safeguarding their assets and reputation.
Challenges and Limitations in Fraud Detection
Despite the advancements in fraud detection using data analytics, several challenges and limitations persist. False Positives and False Negatives are common issues; false positives occur when legitimate transactions are incorrectly flagged as fraudulent, causing unnecessary disruptions, while false negatives happen when actual fraud goes undetected, leading to financial losses.
Data Privacy and Security are significant concerns. The extensive data collection required for effective fraud detection raises questions about user privacy and data protection. Ensuring compliance with data privacy regulations and securing sensitive information from breaches are critical challenges.
Evolving Fraud Tactics also pose difficulties. Fraudsters continuously adapt their methods, often staying ahead of detection algorithms. This constant evolution requires fraud detection systems to be continually updated and refined, which can be resource-intensive.
Integration and Data Quality issues further complicate fraud detection efforts. Combining data from various sources and maintaining high data quality can be challenging. Incomplete or inaccurate data can undermine the effectiveness of fraud detection systems.
Addressing these challenges involves implementing adaptive algorithms, maintaining stringent data security measures, and continually updating fraud detection systems to stay ahead of evolving threats.
Conclusion
Fraud detection and prevention are crucial for protecting organizations and individuals from financial losses and reputational damage. Data analytics plays a pivotal role in identifying and mitigating fraudulent activities through advanced techniques like machine learning and real-time monitoring. While challenges such as false positives, data privacy concerns, and evolving fraud tactics persist, leveraging data-driven insights significantly enhances fraud detection capabilities.
To address these challenges and stay ahead in the field of fraud prevention, pursuing a Data Analytics course in Delhi, Jaipur, Ludhiana, goa, etc, can be highly beneficial. Such courses provide in-depth knowledge of analytical techniques, tools, and technologies essential for effective fraud detection. They offer training in advanced data analytics methods, including machine learning algorithms and data integration strategies, equipping professionals with the skills needed to develop and implement robust fraud detection systems.
By combining the latest analytical techniques with continuous learning, individuals and organizations can enhance their ability to prevent and detect fraud. This proactive approach not only safeguards assets and reputations but also ensures a more secure and resilient operational environment.